If success were measured in enthusiasm, the financial world would be the undisputed leader of artificial intelligence and machine learning. A recent Temenos report found that 66% of global bankers believe new technologies like AI, DevOps and cloud will “drive global banking in the next five years,” and 77% agreed that “unlocking the value of AI will be the difference between winning and losing.”
When it comes to getting AI and ML into production, however, the picture is much less rosy. The study found that only 22% have successfully deployed ML in a production environment so far.
So what’s the reason for the discrepancy? As Fintech Enthusiast Anna Oleksyuk pointed out in a recent article, the problem isn’t the technology itself. Rather, organizations are stymied by a combination of legacy IT infrastructure and a lack of sufficient resources. Here’s why financial organizations struggle to put AI into production — and what you can do about it.
Traditional Digital Transformation Obstacles Are Only Part of the Story
Some of the obstacles to AI adoption will be familiar to anyone who has been in digital transformation at a financial organization. Issues like regulatory compliance, the need to ensure privacy, and security concerns increase the difficulty and cost of putting any new tools into production.
But those sorts of issues aren’t new, particularly for older organizations who have had to update components of legacy systems before. They may increase the complexity and cost of development and deployment, but organizations expect them and more or less understand how to plan for them. It’s the development challenges financial institutions don’t anticipate that cause the most serious problems.
The Difficulty of Building and Deploying AI
The first obstacle businesses run into in AI development is a basic lack of resources and expertise. Building production-ready AI requires specialized knowledge most organizations don’t possess, an integrated development environment, and a lot of work from an IT team that’s already stretched thin. Additionally, financial businesses tend to underestimate how much time it takes to train, supervise, and maintain an AI system once it’s built — particularly organizations that have never deployed one before.
Even teams that have the know-how and tools often don’t have the data. Whether it’s designed to provide actionable insights to financial decision-makers, anticipate swings in the market or look for signs of fraud, AI software thrives on data throughout its entire lifecycle. Even before it goes live, the software needs to be trained with a large, accurate, representative and correctly structured dataset. Unfortunately, most financial institutions aren’t ready from a data governance perspective. Their production dataset is incomplete, incapable of being accessed quickly enough, or otherwise inadequate for their development needs.
Additionally, most financial institutions are sitting on top of a legacy architecture. The systems that execute mission-critical tasks are complicated and brittle, and small changes can have serious unintended consequences. This makes deploying AI much more complicated, with many rounds of testing and refining, which can result in unanticipated delays and cost overruns.
Off-the-Shelf Solutions Are the Best Path to AI Leadership
The financial industry understands the promise of AI. Although adoption is still relatively low, it won’t be for long. By using off-the-shelf AI and ML solutions, you can stay on the cutting edge while focusing on your core competencies.
To learn more about how Bitvore can help you beat the adoption curve without getting bogged down in development, download our case study below!
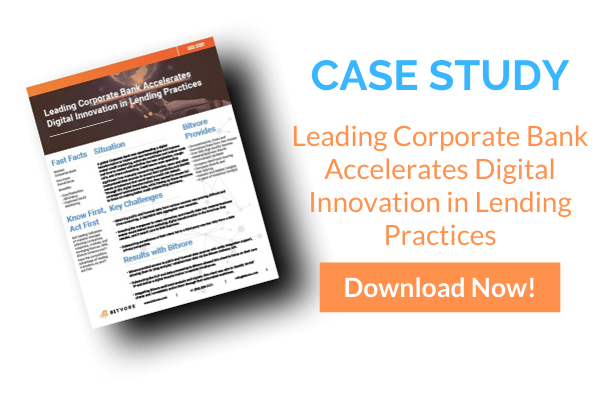