One of the risks of artificial intelligence is that even the most well-intentioned people can write their biases and blind spots into code that makes decisions for a large number of people. In her book, "The Big Nine: How the Tech Titans and Their Thinking Machines Could Warp Humanity," futurist Amy Webb argues that because the nine largest AI companies in the world are led by people who largely share the same culture, background, and education, it becomes harder for them to see and adjust these blind spots when they emerge.
The capstone of Webb's argument is that profit motive makes it inefficient for the Big Nine to fix these systemic issues before they go to market, but we have a different perspective.
AI is a Quieter Revolution Than It Should Be
Right now, AI is mostly about creating efficiencies. Research from McKinsey and Company says that about 63% of survey respondents reported a direct revenue increase from AI, mainly from either pricing their existing products more effectively or using AI to package their existing data for sale. Meanwhile, 44% of respondents generated a revenue increase via cost savings, allowing them to optimize how they use machinery or save money on precursor materials.
For example, you might deploy an AI-powered purchasing software agent on the commodities market. The AI is a better predictor than a human analyst, and it can make decisions faster, and as a result your company can save an average of three cents on the price of pork bellies it uses to make BLT sandwiches. Alternatively, you might use AI to analyze the demographics of the people visiting your BLT sandwich website, realize they have deeper pockets than you thought, and improve revenue by raising your prices.
In either case, the AI isn't doing anything particularly revolutionary, certainly nothing that a data scientist couldn't do with a spreadsheet and a lot of spare time. The advantage is that the AI software is faster than a data scientist and cheaper than a full-time hire.
Problems arise when no one vets this service offering. If your AI solution achieves cost savings by ordering pork bellies from companies that have failed health inspections, for example, then you've got problems.
Our position is this: too many AI companies are looking for use-cases that create profit from the bottom up by effecting these small percentile-level changes. This makes it less likely that companies will vet them and more likely that unanticipated problems will occur. It's not profit motive that's the issue; it's small-picture thinking.
AI Leaders Need to Cut Biases by Thinking Bigger
We asked the leadership here at Bitvore what AI companies should do in order to prevent themselves from falling into the trap of biases and blind spots.
"I think a lot of AI companies out there, they're too focused on here's how you can save money or reduce time-consuming tasks," says Greg Bolcer, Chief Data Officer at Bitvore. "There's not enough focus on building new capabilities. Current AI functionality is like improving resolution on a television, but what AI needs to be doing is building something like a remote control."
"Amy Webb says that large AI companies are comprised of myopic, like-minded groups," says Steve Henning, Chief Marketing Officer at Bitvore. "We're not saying she's wrong, we just think the solution is different. When companies start thinking about larger problems they can solve, they end up bringing more people into contact with AI, increasing the number of perspectives in play and eliminating the problem of myopia in the first place."
Big AI moonshots gain a lot of press, especially if they go wrong. We've all heard horror stories about companies that deploy autonomous school busses illegally, or employment screening applications designed to promote lacrosse players named Jared. The bad press can make AI leadership wary of larger and more impactful projects.
Instead, we argue that big-press failures are outliers. Companies largely know what safe and sustainable AI development looks like. They care enough about quality to delay time to market in service of getting the product right. They're responsible enough to keep humans in the loop before AI can make huge decisions independently. In that light, the safety rails are already in place for AI companies to become more ambitious - and if they do it right, there will be far more than just nine big AI companies deciding our collective future.
Want to learn more about how Bitvore is creating an ambitious AI future of its own? Download our latest white paper: Using Sentiment Analysis on Unstructured Data to Identify Emerging Risk.
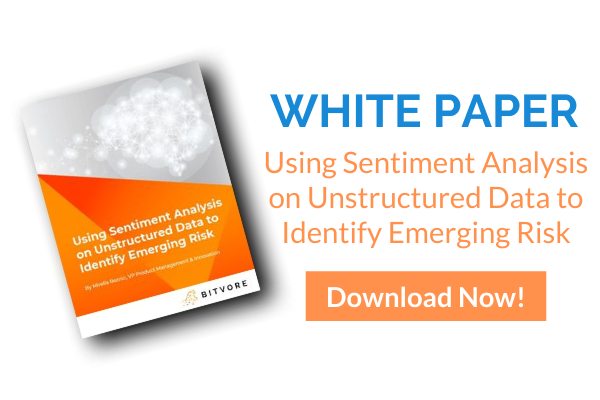